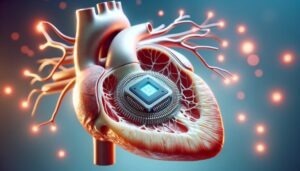
Artificial Intelligence in Transcatheter Aortic Valve Replacement: Its Current Role and Ongoing Challenges
The integration of Artificial Intelligence (AI) into Transcatheter Aortic Valve Replacement (TAVR) is revolutionizing cardiology, offering enhanced patient selection, procedural planning, and post-implantation monitoring. As TAVR becomes a viable option for a broader range of patients with severe aortic stenosis, AI’s role in interpreting medical imaging and developing risk models is increasingly critical. This article delves into AI’s current contributions to TAVR and examines the challenges and future directions of its implementation in ensuring optimized patient outcomes.