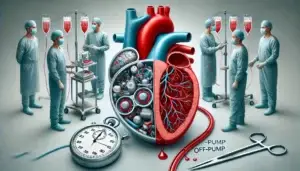
On-Pump or Off-Pump Impact of Diabetic Patients Undergoing Coronary Artery Bypass Grafting: 5-Year Clinical Outcomes
This study evaluates the long-term outcomes of on-pump vs. off-pump coronary artery bypass grafting (CABG) in diabetic patients. A retrospective review of 1,269 patients found that off-pump CABG was associated with a lower 5-year all-cause death rate (4.23% vs. 5.95%) and reduced risks of postoperative stroke and atrial fibrillation. These results suggest potential advantages for diabetic patients undergoing off-pump CABG procedures.