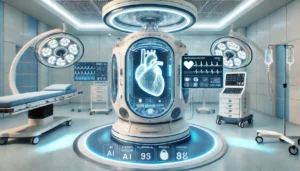
PerfusionGPT: AI-Powered Chatbot for Cardiac Surgery Professionals
PerfusionGPT is an AI-powered chatbot based on ChatGPT-4, specifically designed to provide expert knowledge for perfusionists in cardiac surgery. It serves as a critical resource for both clinical decision-making and educational purposes in the field of perfusion.